Hannah Barker
Ryan Harrison
Elise Milstein
Joe Nudi
Peter L. Levin
Amida Technology Solutions, Inc.
July 2022
Executive Summary
Healthcare CIOs and CDOs must manage an increasing variety of disparate data sources: traditional exchange partners (such as electronic health records [EHRs]), internal sources (such as Payor claims data), and emerging sources (such as patient-generated records from wearables). This superabundance of health data has created an acute demand for data catalogs in healthcare.
Data catalogs are software applications that discover, inventory, and profile data from data lakes, data warehouses, data hubs, and other shared resources. Modern data catalogs often rely on machine learning (ML) to automate the ingestion, translation, and annotation of metadata (the data that describe data). Technical and business stakeholders utilize data catalogs to discover and connect to enterprise assets to gain analytical and operational insights.
Alation, Collibra, and IBM are considered industry leaders. Their standard features include metadata repositories, metadata ingestion and translation, and business glossaries. Data catalogs with intuitive user interfaces are particularly valuable. (Amida’s experience with our Payor, Provider, State, and Federal clients confirm that these are first-in-class tools.)
When healthcare CIOs and CDOs engage professional services to support catalog deployment, they should focus on their vendors’ ability to facilitate governance using a catalog, as well as their clinical domain expertise in organizing a catalog. These considerations are more important than specific (and only slightly differentiated) catalog features such as metadata administration, collaboration features, logging capabilities, and software-enforced governance policy enforcement.
Impact of Data Catalogs
Data catalogs reduce the time to derive value from data and to lower operational costs. They simultaneously ease the burden of adding new data sources and make existing data more accessible to end-users.1 Healthcare enterprises particularly benefit from data catalogs because Patient, Provider, and Payor data become discoverable in a single, searchable application, which facilitates collaboration and enables analytics across organizational silos.2
Healthcare enterprises need a reliable, scalable, comprehensive, and enterprise-wide purview of contextualized data to discover (and curate) information assets. Data catalogs – like old-fashioned library card catalogs – are an inventory of metadata resources; literally, the “data about the data” — or the descriptions and attributes of the underlying taxonomical models, but not their content.3 Card catalogs kept track of information about books that the library possessed or had access to — who wrote them, what they were about, and where to find them. A more-modern example is phone calls: metadata keeps track of who-is-talking-to-whom, and when; data, in this context, are the actual conversations.
Data catalogs organize and classify metadata into three groups:4
• Technical metadata: The form and structure of each data set; examples include the data schema (e.g., attributes, foreign key relationships) and data format (e.g., text, images, JSON)
• Operational metadata: Data lineage, provenance, and quality; for example, the data source and transformations for a derived field
• Business metadata: The definition and meaning of data fields; examples include business names, descriptions, and rules
Data Governance
A CIO or CDO should not select and deploy a data catalog absent an enterprise commitment to ensure organizational data-governance maturity.
Data catalogs support data governance: however, deploying a data catalog in isolation will not fix an enterprise’s underlying governance problems. Even the most sophisticated data catalogs require supervised curation, supported by formal channels of communication and agreement.
Some healthcare enterprises choose centralized curation by designated data owners and data stewards. Others utilize a crowdsourced and distributed team-based curation.5 In either case, data owners or their designees can accept or reject auto-tags within their catalogs, rate the quality of data sets to warn users of potentially noisy (or even unreliable) data, and approve registered data lineage for identification of authoritative sources and provenance for downstream consumers.
Data catalogs also work hand-in-hand with teams that are focused on privacy and security concerns. Metadata assets that contain PHI or PII should be flagged with appropriate indicators for catalog consumers. Most data catalogs have the capability to display sample data for inventoried data sets, and some have features that can assist in the identification and masking of sensitive data elements inside the catalog. This reduces the burden on privacy and compliance teams, and it improves trust between the data catalog team and the owners of the cataloged systems. Further, in the event of an audit or breach risk assessment, organizations may need to demonstrate that sensitive data is masked. Data catalogs that integrate both data masking and data lineage enable tracking of sensitive data and their transformations through the enterprise, and they provide invaluable insight when coupled with core enterprise audit and security tools.6
Data Sources
To support their organization, a CIO or CDO should define what data should be included in the data catalog and then prioritize data sources for onboarding and curation. Data catalogs ingest and consolidate metadata from available data sets via connectors and APIs. In practice, an enterprise data catalog will be populated by a combination of data warehouses, data lakes, and data hubs. Without definition and prioritization, IT and domain-expert staff will become overwhelmed by the number of sources and (poorly defined) data elements involved in the cataloging and curation effort.
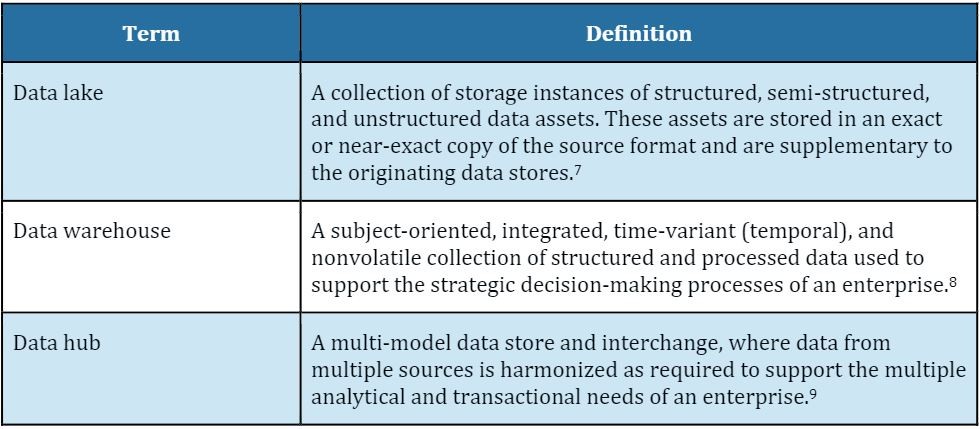
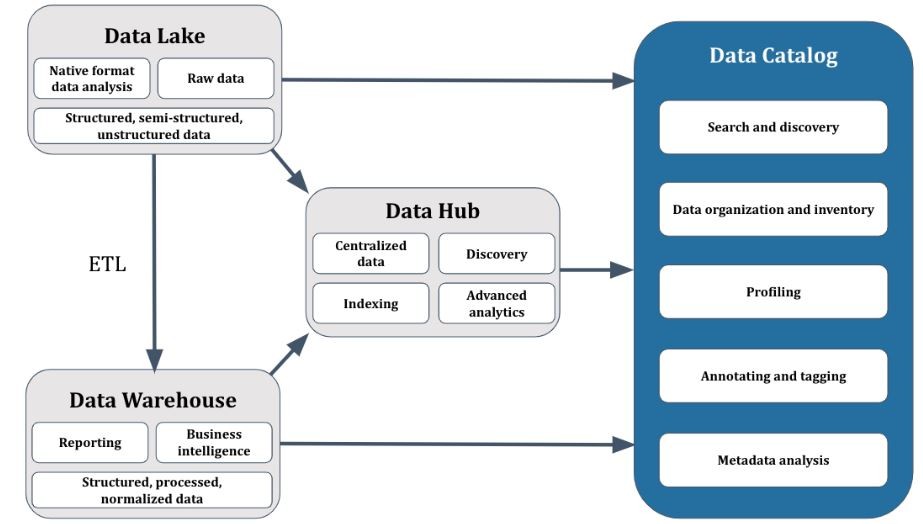
Data Catalog Features
A CIO or CDO should align data catalog features with specific business requirements (with the understanding that the capabilities of the first-in-class data catalogs are broadly similar, and that there is no “killer feature” among the leaders).
Data catalogs offer a suite of solutions for metadata management and are powerful tools the implementation that assist of a Master Data Management (MDM) strategy. Common features of an effective data catalog include an integrated business glossary (which may also be a separate application), data lineage tracking, metadata ingestion, and semantic relationship tagging. In addition, most modern data catalogs include a built catalog )in search function ( to facilitate ad hoc queries across the and integrate with business intelligence tools such as Tableau, Power BI, and Qlik.10
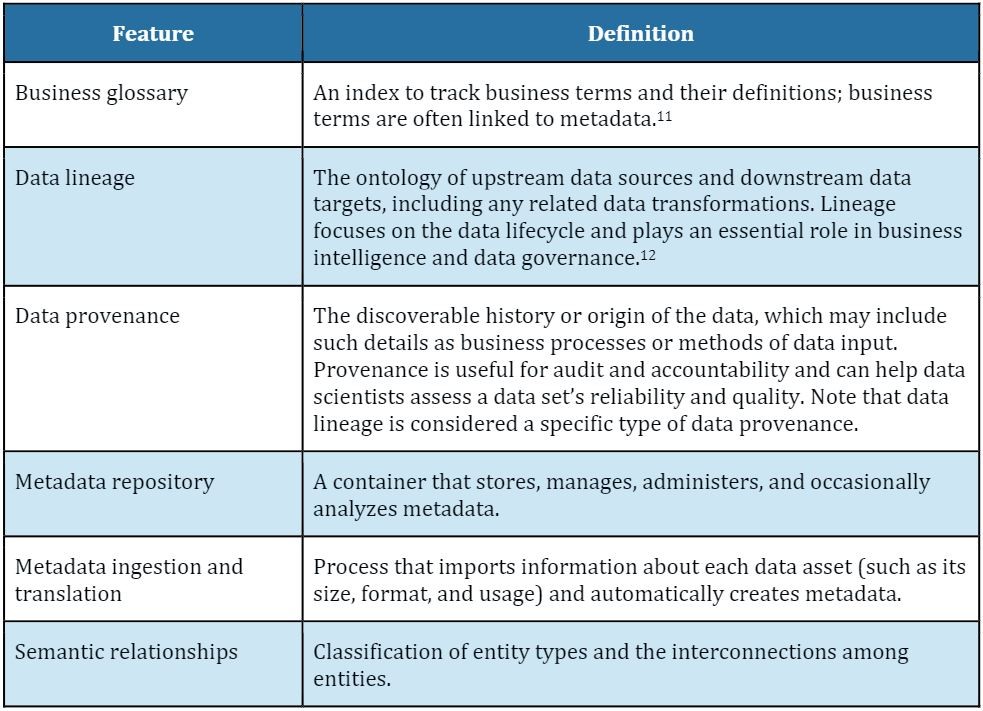
Data Enrichment with Machine Learning
A CIO or CDO should consider automated curation with ML a “nice to have.”
ML data enrichment is used to automatically collect, tag, and annotate metadata, and to establish semantic relationships between data sets. It may reduce the burden on data stewards for repetitive curation tasks. Although marketed heavily by catalog vendors, ML will not rescue a data catalog integration effort that is absent the alignment of governance processes, definition and prioritization of data sources, and core features that map to business requirements.
Once the connected data assets have been scanned, users can interact with their data catalogs and explore annotations, semantic relationships, and profiles (including statistics about table rows and columns).13 Several data catalogs enable users to develop business glossaries directly within the tool, rather than employing a standalone application (or in many enterprises, a Word document). Additionally, some ML data catalogs support import policies and classifications that facilitate the automatic or human-curated linking of business terms to metadata. Healthcare organizations, for example, could build a business glossary with attribute definitions and data lineage for metrics related to re-admission rates and surgical procedures.14 Converged terms derived from the catalog’s glossaries ultimately promote clear communication across the organization and ensure data consistency in analytics and business decisions.15